Zachary W. Ulissi
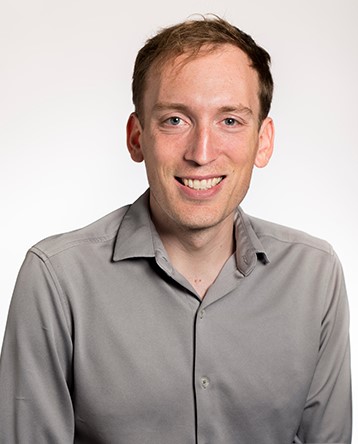
zulissi@meta.com
zulissi@gmail.com
I joined Meta’s Fundamental AI Research lab in 2023 to work on AI for chemistry and climate applications and am located in the SF bay area. I am extremely excited about how AI/ML methods can help many types of quantum chemistry simulations and lead to better materials and catalysts.
In 2024 I am an adjunct professor of chemical engineering at CMU. Prior to 2023 I was an assistant and then associate professor, and in 2023 I was on leave from my position at CMU. I joined Carnegie Mellon University in 2017, after doing my PhD at MIT and post-doc at Stanford. My PhD work at MIT focused on the applications of systems engineering methods to understanding selective nanoscale carbon nanotube devices and sensors under the supervision of Michael Strano and Richard Braatz. I did my postdoctoral work at Stanford with Jens Nørskov where I worked on machine learning techniques to simplify complex catalyst reaction networks, applied to the electrochemical reduction of N2 and CO2 to fuels. At CMU I continued these efforts to model, understand, and design nanoscale interfaces using machine learning and predictive methods to guide detailed molecular simulations.
In my free time, I enjoy the outdoors and used to be a competitive cyclist, though mostly I do bike trips for fun now. I also enjoy cooking and traveling, and have a toddler (Enzo) and a large friendly dog (Laika).
news
Jul 01, 2023 | The OCP Demo website was launched! Check it out at https://open-catalyst.metademolab.com/ |
---|---|
Dec 01, 2022 | AdsorbML, a strategy to use pre-trained GNNs to massively accelerate the adsorbate placement and adsorption energy calculation process, was released on arxiv! |
Jul 01, 2022 | I was promoted to Associate Professor of Chemical Engineering at CMU! |
Jul 01, 2022 | The OC22 dataset and models for oxide electrocatalysts were released. |
Oct 01, 2020 | The OC20 dataset and open ML models were released to the public! |
selected publications
- ML/GNNThe Open DAC 2023 Dataset and Challenges for Sorbent Discovery in Direct Air CaptureACS Central Science, 2024
- ML/GNNCatTSunami: Accelerating Transition State Energy Calculations with Pre-trained Graph Neural NetworksarXiv preprint arXiv:2405.02078, 2024
- ML/GNNOpen materials 2024 (omat24) inorganic materials dataset and modelsarXiv preprint arXiv:2410.12771, 2024
- Catalysis/MLOpen Catalyst Experiments 2024 (OCx24): Bridging Experiments and Computational Models2024
- Catalysis/MLThe Open Catalyst 2022 (OC22) Dataset and Challenges for Oxide ElectrocatalystsACS Catalysis, 2023
- Catalysis/ML